Can AI make A-CDM even better?
Enhancing Capacity Planning in European Aviation: A Game of Tetris
With the increasing demand for air travel, capacity is struggling to keep up. To meet the growing demand for air travel we must find ways to efficiently use our existing capacity. Imagine playing Tetris - the better you use the finite space, the more blocks you can accommodate. That's where Eurocontrol's A-CDM framework comes in.
With the increasing demand for air travel, capacity is struggling to keep up. Capacity refers to airspace, runways, and gates. While airspace can be optimized to increase capacity utilization, expanding runways and gates is expensive and time-consuming. Additionally, many airports are running out of physical space or facing sustainability concerns, limiting their ability to accommodate the expected growth in passengers.
Enhancing Capacity Planning in European Aviation: A Game of Tetris
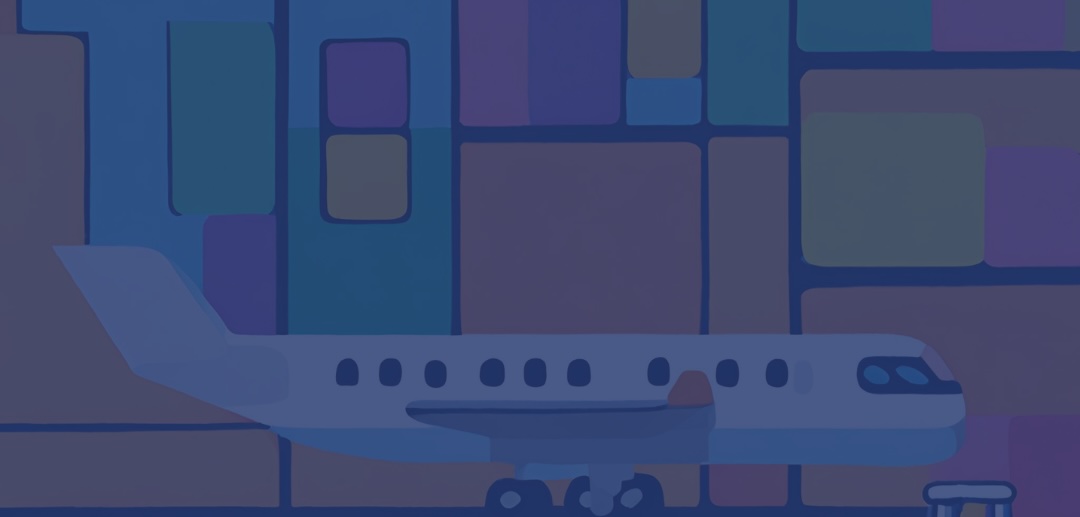
To meet the growing demand for air travel, which is expected to double by 2050, we must find ways to efficiently use our existing capacity. Imagine playing Tetris - the better you use the finite space, the more blocks you can accommodate. That's where Eurocontrol's A-CDM framework comes in.
A-CDM allows us to plan by accurately predicting future conditions. Just like in Tetris, if you know what blocks are coming, you can create the perfect plan. By knowing when aircraft will be at a certain location, whether it's airspace, gates, or runway slots, we can make the best use of the available capacity.
Predictability is key because limited capacity requires buffers to handle surprises. Unpredictability can lead to underutilized capacity, delays, poor passenger experience, and unnecessary carbon emissions and costs.
Consider the case of airspace sectors with maximum aircraft capacity. If delays occur, the sector's capacity goes unused at first and then becomes overwhelmed. This leads to delays, suboptimal speeds, and negative environmental and economic impacts. Similarly, surprise delays during aircraft turnarounds can result in a domino effect, causing delays, carbon emissions, and unhappy passengers.
That's why A-CDM was introduced across major European airports. Ground handlers provide regular updates on turnaround processes, informing all stakeholders of departure readiness. By using these updates to assess flight numbers versus available capacity, optimizations can be made to prevent capacity issues and hold flights at their gates until there are no issues down the line. The goal is to optimize flow, ensuring aircraft can smoothly reach their destinations without queuing, holding, or waiting at any point.
With enhanced capacity planning through A-CDM, European aviation is ready to handle the future growth in air travel efficiently and seamlessly.
Unlocking the Full Potential of A-CDM: Is It Meeting Expectations?
A-CDM has promised significant benefits for all stakeholders involved. Studies have shown positive results, which is encouraging given the investments made to implement this framework. However, we must assess whether it is truly living up to its potential.
At the heart of A-CDM lies accurate estimation by ground handlers of the aircraft's readiness for departure. This estimation is crucial for optimizing operations both at the airport and network levels. Delays in receiving updates from ground handlers can hinder optimization efforts. Unfortunately, in practice, early and accurate updates prove to be a challenge. Ground handlers are often under immense time pressure, juggling multiple tasks with limited resources. As a result, they struggle to gather and digest all the necessary information to accurately estimate the aircraft's ready time.
To truly harness the power of A-CDM, we must address these challenges and ensure that ground handlers have the support they need to provide timely and accurate updates. Only then can we fully realize the potential of this framework.
Looking to improve? Machine learning is the key.
When it comes to analyzing flight data from multiple sources, identifying patterns, and predicting aircraft readiness, AI is the perfect solution. At Assaia, we've discovered that using the existing airport and airline data, we can outperform human estimations of aircraft ready time with our Machine Learning technology.
Unlike humans, computers are unbiased and can focus, with no need to multitask. They continuously analyze the data, detecting even the slightest changes and translating them into predicted impacts on readiness. This leaves human agents free to tackle other vital tasks.
Traditionally, there has been a lack of real-time data during turnarounds, making it a "black box" process. However, with advancements in AI, especially in Computer Vision, we can now measure and capture all relevant turnaround processes. This data is then used to predict aircraft readiness. Combining Computer Vision with Machine Learning sets the standard for AI and data-driven operations.
The best part? Automating aircraft readiness predictions amplifies the benefits of A-CDM by providing more accurate and reliable data for airport and network optimization.