The value of accurate off-block time predictions
Everyone loves a smooth travel experience, right?
Actually those smooth travel experiences are also most cost efficient and sustainable for airports and airlines. Let’s see how predictability can help to create a win-win all around! In this blog post we will explore the intricacies of manually set Off-Block Time (OBT) estimations versus machine generated predictions. We will first look into the importance of OBT before investigating current day Target Off-Block Time (TOBT) performance at several airports. We will then move on to showcase what level of accuracy can be achieved with the use of Machine Learning (ML) technology.
Aircraft at the Centre of the Universe
When considering passenger air travel, the key objective, and therefore the core of passenger experience, is to get from your origin to your destination, safely, on-time and with your baggage (in one piece). However, as it is the aircraft that actually moves the passengers, it is the aircraft’s operation which is critical for the passenger to be successful.
In order for the aircraft to achieve success, it needs a lot of resources. It will need a parking stand at its origin airport, it will need fuel, crew, taxiway, runway, airspace and so on. In that sense, operating aircraft is very much a resource management problem that needs to be solved in the most optimal way. Even the passenger and baggage can be viewed as a resource that needs to be allocated to the right plane at the right time. Please note that optimal resource management always tries to achieve the holy trinity of delivering the best experience, on time and at the lowest possible cost. Different airlines can, however, put a stronger focus on each aspect. One might focus more on cost (think Ryanair), experience (think KLM) or punctuality (think JAL Airlines).
The Power of Proactive Information
For the purpose of this article, let’s define optimization as the allocation of resources to an aircraft in the best possible way to achieve a pre-defined goal. In order to optimize, information on many different variables is required. For example, if you do not know how long boarding a Boeing 737-800 aircraft takes, how can you plan turnarounds, departure, arrivals and crew hours?
The very interesting thing about optimization is that it typically happens on multiple time horizons. It all starts with planning, which consists of creating an ideal schedule of how you would like things to happen. As we approach the day of operation, reality kicks in and starts changing the value of the variables you have used for planning. However, if you are aware of these changes in time, you can act proactively and ensure successful execution of your operations.
For example, if you know in time that there is an IT failure which means boarding will take twice as long as normal, you can still make a gate change. This is when we get into the domain of operations management which typically involves acting and adapting quickly to changes that are happening to the way you planned your operations. The earlier and the more accurate your information is, the better you will be able to achieve success.
The Importance of Accurate OBT
Building on the previous sections, information about the aircraft is especially important as all other resources will need to be synchronized with it. The stand needs to be available as the aircraft arrives, the fueller needs to come to the right stand at the right time, etc. It is for this very reason that the Airport Collaborative Decision Making (A-CDM) framework focuses on different milestones (take-off times, landing times, etc) which are all related to the aircraft. For successful operations management it is important to have accurate and proactive information on these variables in order to adapt and make sure you achieve your predefined goals.
The figure below depicts a typical implementation of the A-CDM process.
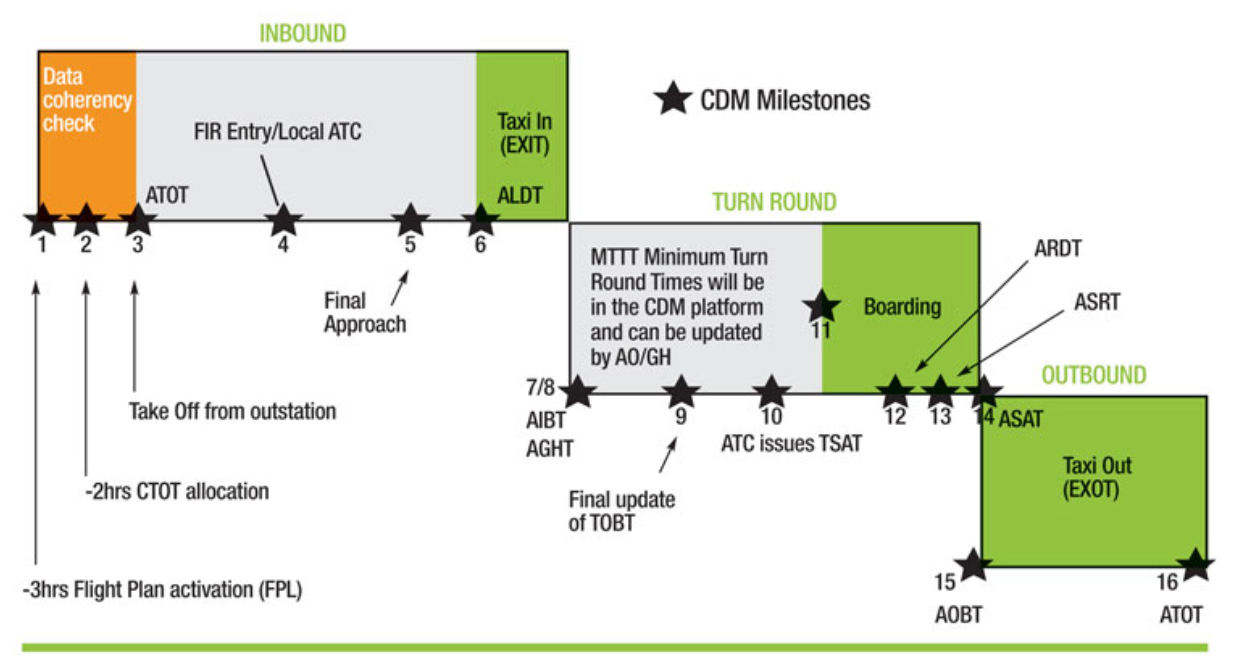
Before we continue, let us consider the relative importance of all these variables. First, some of the variables will be more uncertain than others. It is especially important to have proactive information on the uncertain variables (which have a high degree of variance) because they have the highest likelihood to impact your predefined plan.
Second, the variables on resources that are more scarce, or difficult to activate on short notice, are more important than those which are abundantly available. For example, if you have plenty of aircraft stands available, it is less critical than if you would have only a few aircraft stands available.
Finally, there are some variables which are more fundamental than others. Those variables which are on the critical path and which many other things depend on, are more important than those that operate in isolation. As an example, let’s consider cleaning during aircraft turnaround. It is critical as no boarding can happen before cleaning has finished. This is contrary to, for example, baggage loading which does not have any other events depending on it.
Now let us revert back to the topic of OBT. The OBT is a critical moment as on one side it depends on many factors, while on the other hand it also influences everything that happens afterwards. The fact that it is so dependent means that in practice it is a variable with a high uncertainty (almost no flights leave exactly on the planned moment). Furthermore, consider the fact that basically everything that is planned around an aircraft for an entire day is dependent on the OBT of the first departure of that day. This is why airlines focus so much on the ‘first wave’ of operations. If they manage this ‘first wave’ well, it is a solid base for the rest of the day. If the ‘first wave’ is delayed, there will be knock-on effects all day long. Especially in busy schedules there is no time to recuperate from these effects. Lastly, some of the most scarce resources like runway and airspace capacity are allocated to the aircraft based on when it will go off-block.
Especially with regards to this last point it is interesting to look at the A-CDM framework in a bit more detail. A schematic overview of the A-CDM process is shown below. You can see that in order to manage the usage of airspace and airport capacity, Eurocontrol and local Air Traffic Controllers (ATC) optimize the use of these resources based on when they expect the aircraft to need it.

TOBT is the cornerstone of these efforts but therefore also constitutes a single source of weakness. If the TOBT is inaccurate, the aircraft will be expected on the runway and in different airspace sectors at the wrong time. This means that when the aircraft is not there, precious capacity is lost. It also means that the aircraft will need this capacity later when it’s not planned and possibly unavailable. This leads to delays.
Today’s OBT Performance
In today's operations, the source of OBT information is typically an airline or ground handling agent who is responsible for turnaround coordination. This can either be someone who is at the aircraft overseeing the turnaround or someone in a control centre remotely managing turnaround operations.
Before we share some results from our studies on TOBT accuracy, it is important to note that estimating off-block times is typically just one out of many tasks for the ground handling agents. Furthermore (especially when turnarounds are remotely managed), there is little real time turnaround data available. For example: whether or not a fueller is actually at the aircraft or whether it is late, is often unknown. For this reason the turnaround is often referred to as a black box and makes it very hard to create accurate estimations of the OBT. Lastly, ground handlers are operating with extremely thin margins and are therefore usually under a lot of time pressure to perform their tasks.
First of all, let’s look at the number of flights for which TOBT is actually set before we investigate accuracy. We investigated four medium / large European hub airports for which we can see somewhat different results. Airport 3 has a significantly lower percentage of flights with TOBT than all others. Furthermore, we can also see that the average TOBT inaccuracy is rather consistent at four to five minutes (we define accuracy as the difference between TOBT or POBT and AOBT).

Looking only at the average accuracy, however, does not tell us the full picture. We also need to consider the variance because it could be the case there are a lot of outliers which do not affect the average inaccuracy but are still very costly cases. The variance is defined as the standard deviation which means 68% of all flights have a TOBT inaccuracy of the average + /- one standard deviation (for example, for airport 1, 68% of all flights have a TOBT accuracy between -3 and 13 minutes from AOBT). Note that this also means that for 32% of all flights the TOBT accuracy is larger than -3 and 13 minutes.
Cost of Bad Performance
Before we move on to show you the accuracy of Machine-Learning based OBT predictions, let’s consider for a minute the cost associated with poor OBT accuracy. Because OBT accuracy is at the fundament of optimal aircraft operations it has many downstream effects which are not always easy to explain and/or quantify. In order to avoid turning this blogpost into a book, we will only focus on three implications in more detail.
TSAT Window Adherence
As part of the A-CDM framework, each departing aircraft receives a slot. This slot is a time reservation for airport and airspace capacity. In order to ensure that the aircraft adheres to this slot it receives a Target Start-Up Approval Time (TSAT) which is the time around which the aircraft may request to be pushed back and start its engines. This time is primarily based on the TOBT created by the ground handler. The pilot must request start-up +/- 5min from the TSAT. If the pilot misses this window, it loses its slot and will only be accommodated at the next available free slot.
If we look at the data from our four airports we can see that flights which depart within their TSAT window are significantly less delayed than flights that missed their TSAT window.
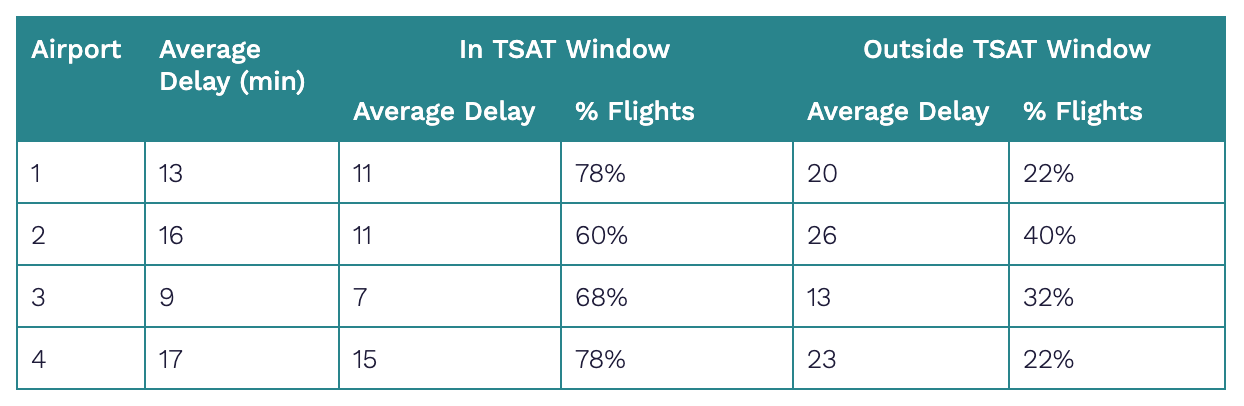
As the TSAT is largely based on the TOBT, it pays off to have a more accurate TOBT because you will have a better ‘fitting’ TSAT window that will result in less departure delays. We can see that for airport 2 the average delay difference is as much as 15 minutes. Furthermore, we can also see that at airport 2 as much as 40% of all flights miss their TSAT window. Since these are all medium / large hub airports we are talking about tens of thousands of flights per year for which an average of 9 minutes can be saved. At the cost of EUR 81 per delay minute, these are multiple millions in potential annual savings to any airline.
Air Traffic Flow Management delays
As in Europe Eurocontrol regulates network capacity, we can see how many flights are affected by capacity shortages. These are the flights for which Eurocontrol issues an artificial delay which means the aircraft has to wait at the gate because an enroute or destination capacity shortage is expected. These delays are referred to as Air Traffic Flow Management (ATFM) delays. For reference: Airports 1 and 4 had 18% and 21% of their outbound flights regulated with an average delay of 19 and 21 minutes.
From these figures it is clear that more capacity would lead to less regulation and thus less delays. We already saw that if a flight misses its slot, it will leave capacity unused and will need more capacity later on. Therefore, more accurate TOBT which results in better ‘fitting’ TSAT windows leads to less slots being missed, less capacity unused and therefore less capacity related delays. Furthermore, in order to account for the inaccuracy that is present in the system today, buffers are built into the departure slot planning. While buffers serve their purpose by preventing situations where demand is bigger than capacity, it also artificially reduces capacity. More accurate TOBTs would reduce the uncertainty and variance in the system and would therefore allow for smaller buffers and thus more capacity.
Airport Capacity
Lastly let's consider airport capacity. If an inaccurate TOBT causes a flight to miss its TSAT window, it means that it is occupying an aircraft stand longer than anticipated and necessary. Furthermore, it also leaves a runway slot unused while needing another one later on. And just as with airspace capacity management, airports and air traffic controllers are using inefficient buffers in their plannings in order to accommodate the variance that is an everyday reality. As many airports are capacity constrained, these situations might artificially lower the airport’s capacity and therefore reduce the number of flights it can accommodate. A typical widebody or narrowbody flight brings an airport EUR 10,000 or EUR 6,000 in aeronautical and commercial revenues. Therefore, improving TOBT accuracy, reducing delays and reducing planning buffers has a direct potential impact on airports’ revenues.
Machine Learning OBT Predictions
So how can we improve TOBT accuracy? Let us introduce Machine Learning (ML) technology. ML is a subfield in the domain of Artificial Intelligence and aims at using large quantities of data to detect subtle patterns and predict values. Using ML technology to predict the OBT is a much better approach than the current day manual alternative.
First, a ML algorithm is dedicated to its task, it cannot be distracted and works continuously to create the best prediction possible. Second, an algorithm is much better able to consume vast amounts of data from various sources and detect subtle patterns.
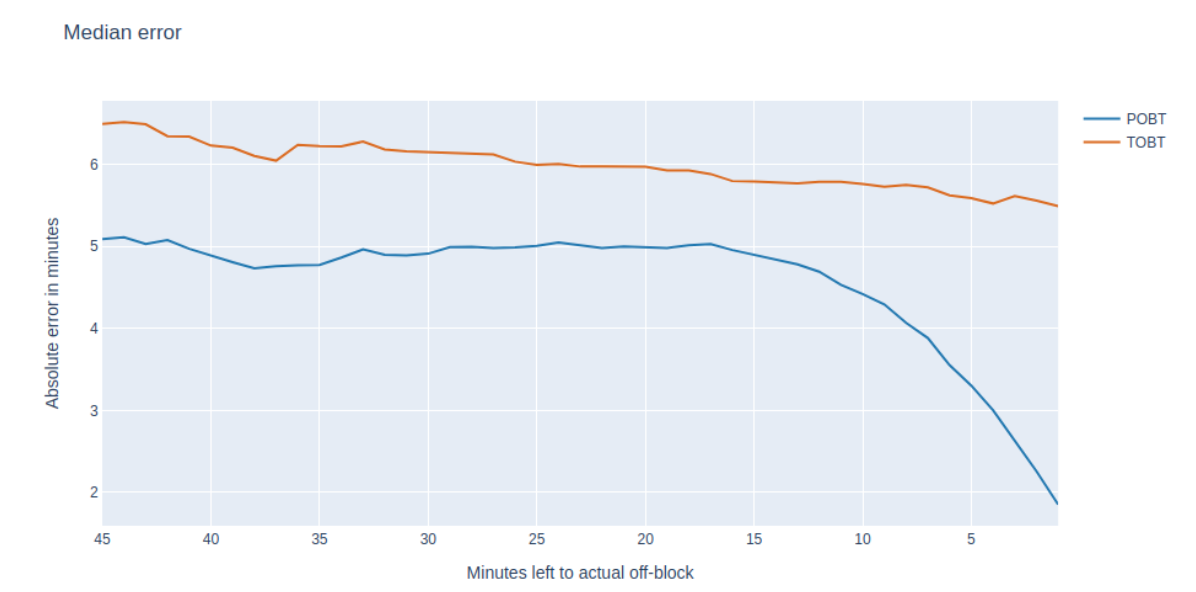
Please see the graph for a comparison of the accuracy of Assaia’s OBT predictor (blue line) versus the current TOBT (orange line) for Airport 1. We can see that the mean error of the predictor is ± 1 minute lower for the predictor than the TOBT up to 15 minutes before actual off-block time. From that point onwards the predictor’s supremacy over the human generated TOBT increases to being almost twice as accurate shortly before the actual off-block moment.
As mentioned earlier, besides the average error, it is important to look at the variance of the TOBT versus the predictor. In the next graph, the red and blue lines show the TOBT and predictor standard deviations.
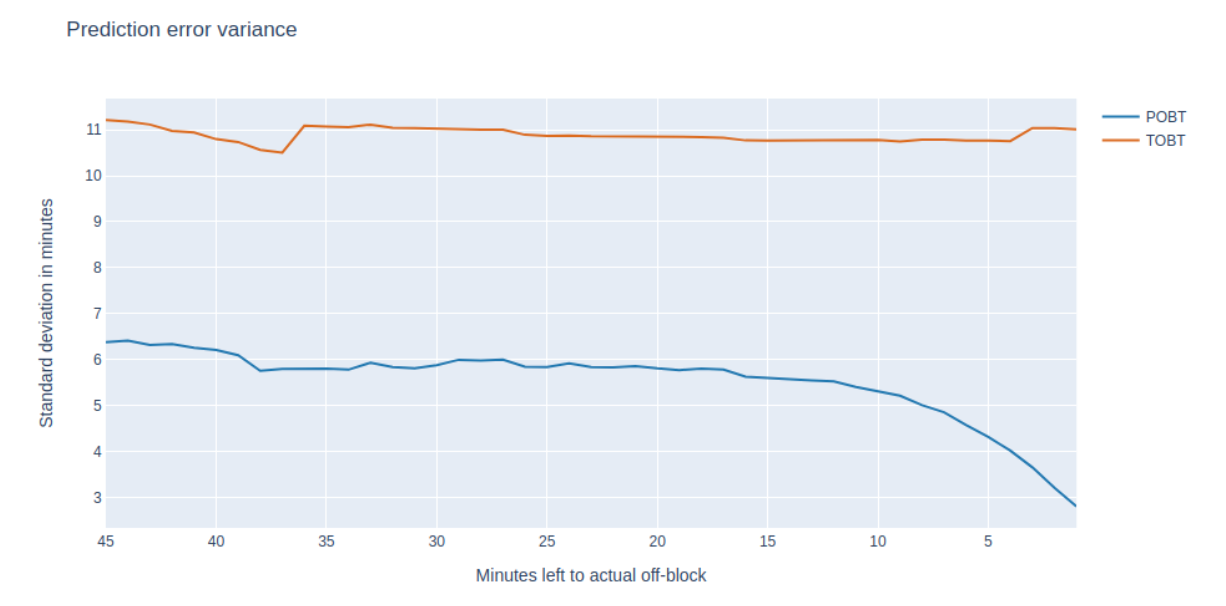
It is very clear that the machine generated prediction provides a much more stable (predictable) performance compared to the TOBT. These results imply that 68% of all flights will have a TOBT which is more than 11 minutes bigger or smaller than the average TOBT error. The remaining 32% of all flights will have an even larger deviation from the average error. We can also see that this large variance of TOBT accuracy does not improve as the actual off-block time comes closer.
In contrast the OBT predictor is much more stable with almost half the variance of the TOBT. This means that for 68% of all flights, the POBT accuracy lies within 6 minutes of the average POBT error.
To highlight the magnitude of these differences let us go back to our 4 airports which we looked at earlier. For the sake of this analysis we will assume that the TOBT is the sole determinant of the TSAT window. This means that if, 5 minutes before departure the predictor is 2,5 minutes more accurate than the TOBT, we can create a new TSAT window for all flights which currently miss their TSAT window by 2,5 minutes. For the 4 airports under study this would affect, on average, 13% of all their flights with an average delay reduction of 3 minutes! We can see that predictability is key to reducing delays while also making better use of existing capacity.
How it Works
The more data the predictor has available to it, the more accurately it can predict. However, only using data that airports already have available today (mainly AODB data), can already provide a large performance improvement over manually estimated OBTs. To reach the ultimate level of accuracy it is obviously also important to have real time information on what is happening during aircraft turnaround. Therefore, the best results can be achieved in combination with accurate real time turnaround data.
The implementation of an OBT predictor does not necessarily change the TOBT practices at an airport. In most cases the manually generated TOBT is a very valuable input for the OBT predictor. Therefore, when implementing the OBT predictor, we do not change the operational A-CDM process. We only re-route the manually generated TOBT to our OBT predictor and forward the output from the predictor to the airport’s AODB.
Assaia’s OBT algorithms have been trained on datasets from airports and airlines all over the world to learn the inherent patterns of airport and aircraft operations. If you are interested to learn more about the potential in your specific case, please reach out to us. We are happy to offer you a free TOBT / POBT comparison based on data that you can make available to us.
Christiaan Hen has spent his entire career in the aviation industry. He worked for over 8 years at Amsterdam Airport Schiphol in different roles like Airport Development, Capacity Management and Operations Management. The last three years at Schiphol Christiaan was Head of Innovation. At the end of 2018 Christiaan joined Assaia to become their Chief Customer Officer. In this role he is primarily responsible for ensuring that Assaia customers derive maximum value from the Apron AI product. Christiaan uses his extensive knowledge of the aviation industry to help make the apron more efficient, safer and more sustainable.